The Telecom Revenue-Growth Newsletter
Our coveted monthly newsletter compiles vetted insights on creating effective sales and distribution chains that fulfil telcos' revenue goals. That, and a lot more.
Here’s a list of what will be covered in this blog:
Introduction
The telecom industry stands at a crossroads. As demand for faster, smarter, and more efficient services soars, telcos are under increasing pressure to innovate. Enter Artificial Intelligence (AI), a game-changing force that promises to revolutionize everything from network management to customer engagement. But while AI in telecom offers immense potential, the journey toward adoption is far from straightforward. For telecom CXOs, AI represents a double-edged sword.
On one hand, it offers unparalleled opportunities for enhancing operational efficiency and driving revenue growth. On the other, the hurdles of integration, talent shortages, and financial constraints loom large. This blog unpacks the most pressing AI challenges telecom leaders face and explores strategic solutions to help overcome these obstacles.
The Data Conundrum: Integration and Management
Telcos generate and handle massive amounts of data—whether from customer interactions, network performance metrics, or IoT devices. However, most of this data remains siloed across different platforms, hindering the full potential of AI-driven analytics. To derive meaningful insights and actionable outcomes, telecoms need to unify these data sets into a cohesive framework that AI can effectively leverage.
Take Vodafone’s AI-powered virtual assistant TOBi, for example. It highlights how AI can be used to streamline customer service, managing queries and resolving issues in real time. However, this success hinges on robust data integration. In fragmented data environments, AI struggles to deliver value. Without addressing these integration challenges, telecom leaders risk suboptimal AI implementations that fall short of their transformative potential.
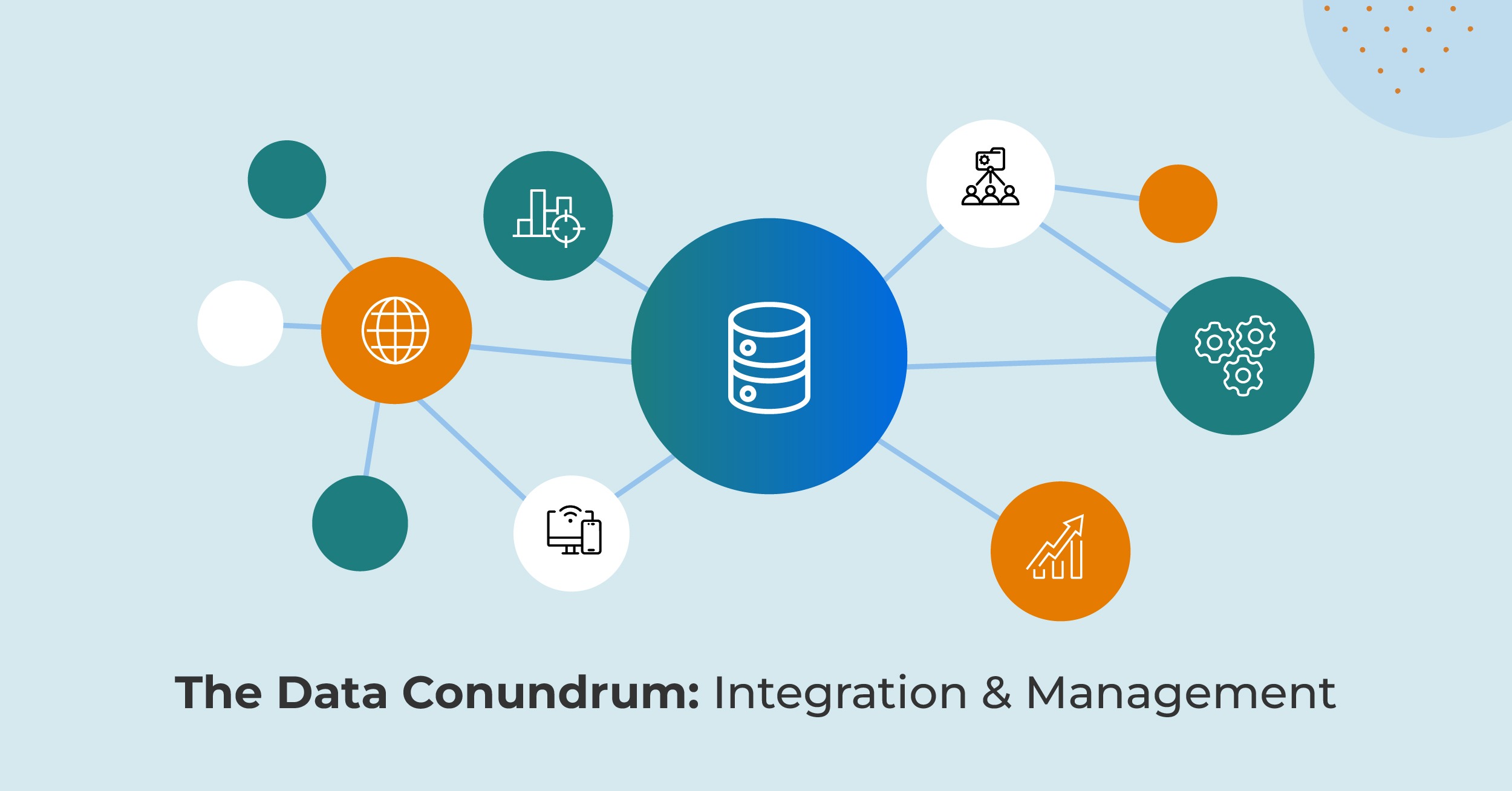
AI-driven solutions play a major role in overcoming challenges faced by MNOs in streamlining retail value management. Utilizing advanced machine learning algorithms allows telecom providers to optimize their daily operations, ensuring more efficient processes and better resource allocation. Such improvements lead to both operational efficiency and cost savings across various business segments.
Moreover, ensuring data accuracy and consistency across multiple platforms remains a challenge, particularly in fragmented data environments. This hinders AI’s ability to deliver actionable insights.
Challenges of Legacy Systems
Truth be told, MNOs are often shackled by legacy infrastructure that was never designed to accommodate AI. For AI to be successfully integrated into existing systems, significant investments are needed to modernize outdated technology. This challenge is particularly acute for operators with sprawling networks and diverse technology stacks, where the cost and complexity of overhauling legacy systems can be prohibitive.
But modernization doesn’t always require a complete overhaul. Many operators have opted for a phased approach, focusing on incremental AI adoption to avoid large-scale disruptions. For example, AI can be used to optimize sales and distribution processes without overhauling the entire backend system. This enables companies to achieve quick wins while paving the way for more comprehensive AI adoption in the future.
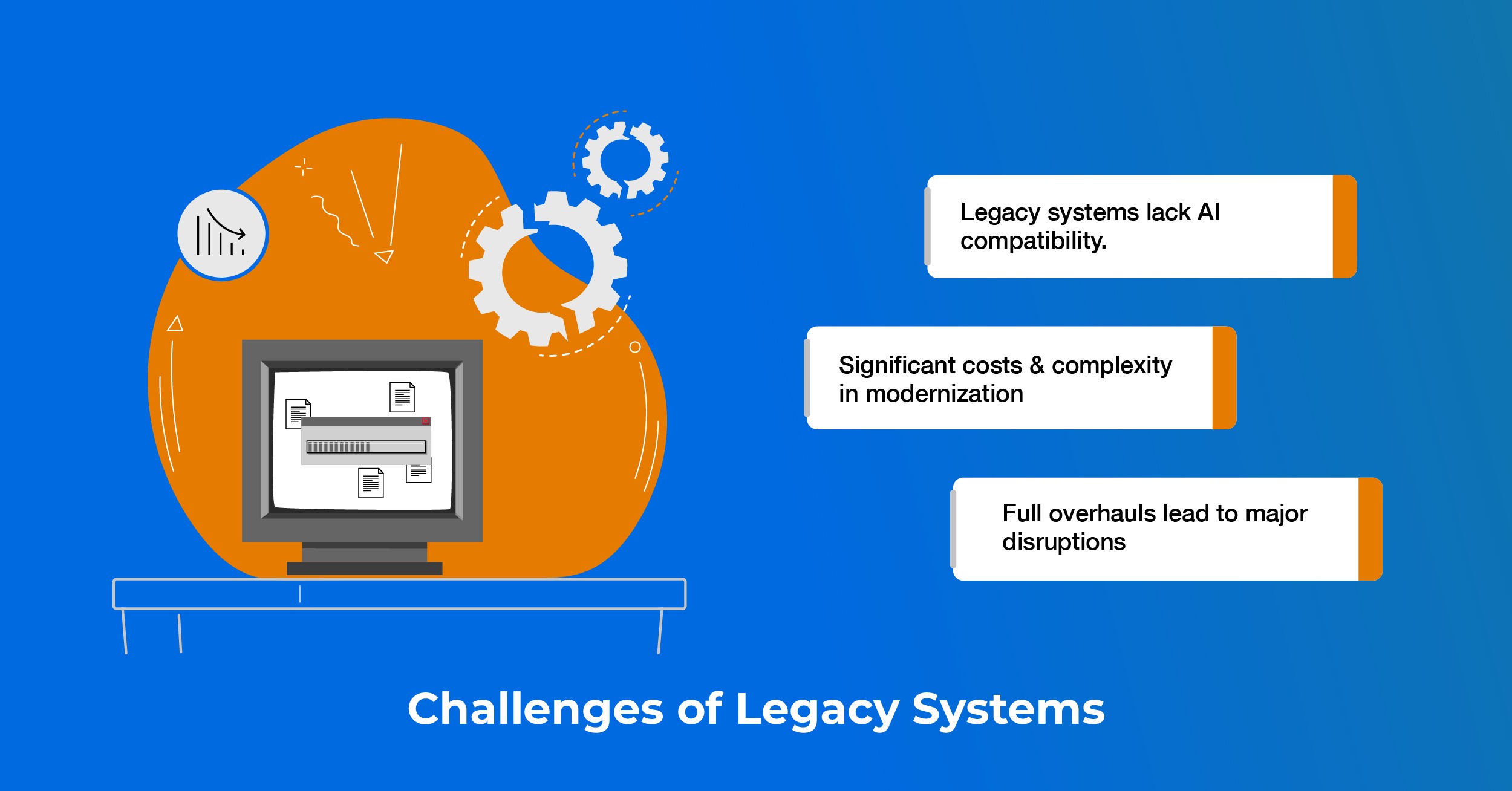
AI is rapidly cementing its place in optimizing sales operations, from real-time forecasting of stock shortages to enabling proactive measures that mitigate disruptions. These capabilities are vital for modernizing sales and distribution processes, allowing businesses to adapt without requiring a complete overhaul of their existing infrastructures. Features such as automated alerts further help by highlighting critical trends and providing actionable insights to adjust strategies swiftly and effectively.
Financial and Operational Constraints
AI adoption is not just a technological challenge; it’s also a financial one. The costs associated with implementing AI in telecom can be prohibitive, especially for smaller or regional telcos. These costs include not only the initial investment in AI technologies but also the ongoing expenses related to system maintenance and upgrades. Financial limitations can also slow down AI adoption, forcing operators to prioritize certain areas while delaying others.
Operationally, integrating AI into telecom processes can cause disruptions, particularly if the transition is not carefully managed. Telecom companies must strike a balance between maintaining service continuity and adopting new AI-driven processes. The key is to choose a strategy that aligns with the company’s operational goals and financial health while ensuring long-term scalability.
The Skills Gap
The telecom sector faces a pronounced shortage of skilled professionals capable of implementing and managing AI systems. However, AI and automation offer a solution by reducing the reliance on specialized human expertise. As AI technology evolves, the industry can leverage AI-driven tools and automated processes to manage complex systems, streamline operations, and drive innovation with fewer resources.
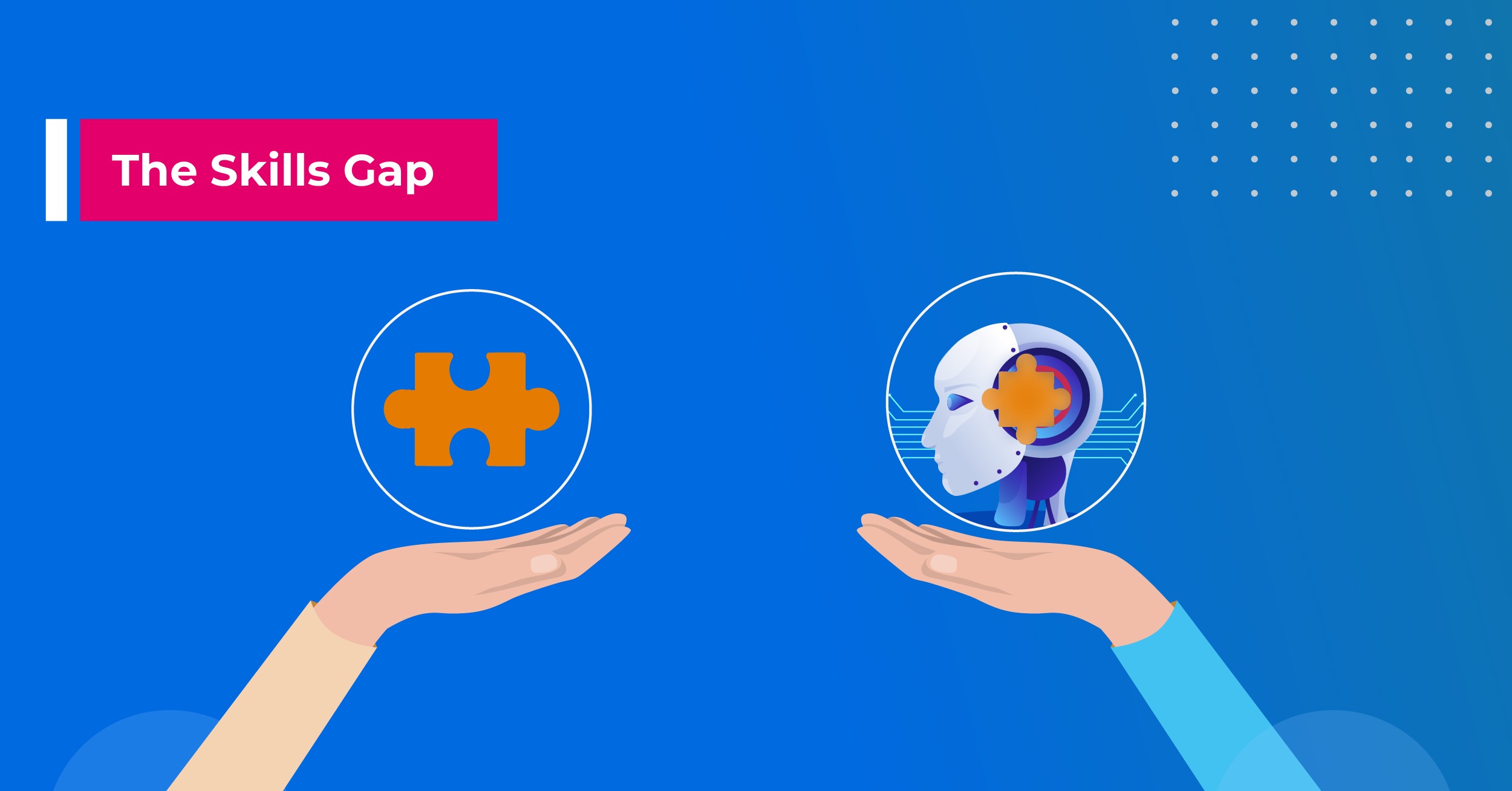
For instance, AI-driven network management systems can autonomously monitor performance, predict outages, and even optimize bandwidth, reducing the need for hands-on intervention. By automating these critical functions, telecom operators can focus their human resources on strategic initiatives rather than routine maintenance.
Privacy and Security Concerns
AI-driven automation offers MNOs great transformative potential, but it also opens new avenues for cyber threats. As AI systems evolve, they can inadvertently create vulnerabilities, exposing sensitive customer data, network infrastructure, and operational metrics to risk. AI-powered malware can adapt to bypass traditional security defenses, making real-time threat detection essential.
As AI becomes more embedded in telecom operations, ensuring data integrity across platforms and robust security frameworks will be essential for protecting these systems from increasingly sophisticated cyber threats.
Final Thoughts
AI holds transformative potential for the telecom industry, offering the ability to streamline operations, enhance customer experiences, and drive innovation. However, the road to successful adoption is filled with challenges, from integrating data across legacy systems to managing financial, operational, and talent constraints.
By approaching AI adoption strategically – whether through phased integration, or leveraging AI-driven security solutions – MNOs can unlock significant value and future-proof their businesses. The key lies in balancing immediate needs with long-term scalability, ensuring that AI is not just implemented but fully integrated into the organization’s core processes.
For CXOs, navigating these challenges isn’t just about adopting new technology—it’s about building a future-ready organization capable of thriving in a digital-first world.